Guessing and Nature of Multidimensionality Matter: A Cautionary Note on the Use of Fit Indices to Assess Unidimensionality of Binary Data
Article ID: 356
DOI: https://doi.org/10.30564/jiep.v1i1.356
Abstract
Keywords
Full Text:
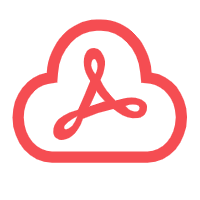
References
[1] Ackerman, T. A. (1989). Unidimensional IRT calibration of compensatory and noncompensatory multidimensional items. Applied Psychological Measurement, 13(2), 113-127.
[2] Dorans, N. J., & Kingston, N. M. (1985). The effects of violations of unidimensionality on the estimation of item and ability parameters and on item response theory equating of the GRE verbal scale. Journal of Educational Measurement, 22(4), 249-262.
[3] Kirisci, L., Hsu, T. C., & Yu, L. (2001). Robustness of item parameter estimation programs to assumptions of unidimensionality and normality. Applied Psychological Measurement, 25(2), 146-162.
[4] Zhang, B. (2010). Assessing the accuracy and consistency of language proficiency classification under competing measurement models. Language Testing, 27(1), 119-140.
[5] Stout, W. (1987). A nonparametric approach for assessing latent trait unidimensionality. Psychometrika, 52(4), 589-617.
[6] Green, S. B., Lissitz, R. W., & Mulaik, S. A. (1977). Limitations of coefficient alpha as an index of test unidimensionality. Educational and Psychological Measurement, 37(4), 827-838.
[7] Hattie, J. (1985). Methodology review: assessing unidimensionality of tests and items. Applied Psychological Measurement, 9(2), 139-164.
[8] Raykov, T., & Pohl, S. (2013). Essential unidimensionality examination for multicomponent scales: an interrelationship decomposition approach. Educational and Psychological Measurement, 73(4), 581-600.
[9] Stout, W. F. (1990). A new item response theory modeling approach with applications to unidimensionality assessment and ability estimation. Psychometrika, 55(2), 293-325.
[10] Bartolucci, F. (2007). A class of multidimensional IRT models for testing unidimensionality and clustering items. Psychometrika, 72(2), 141.
[11] Bejar, I. I. (1980). A procedure for investigating the unidimensionality of achievement tests based on item parameter estimates. Journal of Educational Measurement, 17(4), 283-296.
[12] Christensen, K. B., Bjorner, J. B., Kreiner, S., & Petersen, J. H. (2002). Testing unidimensionality in polytomous Rasch models. Psychometrika, 67(4), 563-574.
[13] Debelak, R., & Arendasy, M. (2012). An algorithm for testing unidimensionality and clustering items in Rasch measurement. Educational and Psychological Measurement, 72(3), 375-387.
[14] Heene, M., Kyngdon, A., & Sckopke, P. (2016). Detecting violations of unidimensionality by order-restricted inference methods. Frontiers in Applied Mathematics and Statistics, 2, 3.
[15] McDonald, R. P., & Mok, M. M. C. (1995). Goodness of fit in item response models. Multivariate Behavioral Research, 30(1), 23-40.
[16] Reise, S. P., Morizot, J., & Hays, R. D. (2007). The role of the bifactor model in resolving dimensionality issues in health outcomes measures. Quality of Life Research, 16(1), 19-31.
[17] Weng, L. J., & Cheng, C. P. (2005). Parallel analysis with unidimensional binary data. Educational and Psychological Measurement, 65(5), 697-716.
[18] Zhang, J., & Stout, W. (1999). The theoretical DETECT index of dimensionality and its application to approximate simple structure. Psychometrika, 64(2), 213-249.
[19] Millsap R. E. (2011). Statistical Approaches to Measurement Invariance. Taylor and Francis Group: New York.
[20] Bollen, K. A. (1989). Structural equations with latent variables. New York: Wiley.
[21] Kamata, A., & Bauer, D. J. (2008). A note on the relation between factor analytic and item response theory models. Structural Equation Modeling, 15(1), 136-153.
[22] Knol, D. L., & Berger, M. P. (1991). Empirical comparison between factor analysis and multidimensional item response models. Multivariate Behavioral Research, 26(3), 457-477.
[23] Luo, Y. (2018). A short note on estimating the testlet model with different estimators in Mplus. Educational and Psychological Measurement, 78(3), 517-529.
[24] Luo, Y., & Dimitrov, D. M. (2018). A short note on obtaining point estimates of the IRT ability parameter with MCMC estimation in Mplus: how many plausible values are needed? Educational and Psychological Measurement.
[25] Takane, Y., & De Leeuw, J. (1987). On the relationship between item response theory and factor analysis of discretized variables. Psychometrika, 52(3), 393-408.
[26] Hu, L. T., & Bentler, P. M. (1999). Cutoff criteria for fit indexes in covariance structure analysis: Conventional criteria versus new alternatives. Structural Equation Modeling, 6(1), 1-55.
[27] Bonifay, W. E., Reise, S. P., Scheines, R., & Meijer, R. R. (2015). When are multidimensional data unidimensional enough for structural equation modeling? An evaluation of the DETECT multidimensionality index. Structural Equation Modeling, 22(4), 504-516.
[28] Beauducel, A., & Wittmann, W. W. (2005). Simulation study on fit indexes in CFA based on data with slightly distorted simple structure. Structural Equation Modeling, 12(1), 41-75.
[29] Fan, X., & Sivo, S. A. (2005). Sensitivity of fit indexes to misspecified structural or measurement model components: Rationale of two-index strategy revisited. Structural Equation Modeling, 12(3), 343-367.
[30] Fan, X., & Sivo, S. A. (2007). Sensitivity of fit indices to model misspecification and model types. Multivariate Behavioral Research, 42(3), 509-529.
[31] Marsh, H. W., Hau, K. T., & Wen, Z. (2004). In search of golden rules: Comment on hypothesis-testing approaches to setting cutoff values for fit indexes and dangers in overgeneralizing Hu and Bentler's (1999) findings. Structural Equation Modeling, 11(3), 320-341.
[32] Yuan, K. H. (2005). Fit indices versus test statistics. Multivariate Behavioral Research, 40(1), 115-148.
[33] Huggins-Manley, A. C., & Han, H. (2016). Assessing the sensitivity of weighted least squares model fit indexes to local dependence in item response theory models. Structural Equation Modeling, 24(3), 331-340.
[34] Nye, C. D., & Drasgow, F. (2011). Assessing goodness of fit: Simple rules of thumb simply do not work. Organizational Research Methods, 14(3), 548-570.
[35] Yu, C. Y. (2002). Evaluating cutoff criteria of model fit indices for latent variable models with binary and continuous outcomes (Doctoral dissertation, University of California Los Angeles).
[36] McNeish, D., An, J., & Hancock, G. R. (2017). The thorny relation between measurement quality and fit index cutoffs in latent variable models. Journal of Personality Assessment.
[37] Hancock, G. R., & Mueller, R. O. (2011). The reliability paradox in assessing structural relations within covariance structure models. Educational and Psychological Measurement, 71(2), 306-324.
[38] Stone, C. A., & Yeh, C. C. (2006). Assessing the dimensionality and factor Structure of multiple-choice exams an empirical comparison of methods using the multistate bar examination. Educational and Psychological Measurement, 66(2), 193-214.
[39] Tate, R. (2003). A comparison of selected empirical methods for assessing the structure of responses to test items. Applied Psychological Measurement, 27(3), 159-203.
[40] Yeh, C. C. (2007). The effect of guessing on assessing dimensionality in multiple-choice tests: A Monte Carlo study with application (Doctoral dissertation, University of Pittsburgh).
[41] Bolt, D. M., & Lall, V. F. (2003). Estimation of compensatory and noncompensatory multidimensional item response models using Markov chain Monte Carlo. Applied Psychological Measurement, 27(6), 395-414.
[42] Hattie, J., Krakowski, K., Jane Rogers, H., & Swaminathan, H. (1996). An assessment of Stout's index of essential unidimensionality. Applied Psychological Measurement, 20(1), 1-14.
[43] Svetina, D. (2013). Assessing dimensionality of noncompensatory multidimensional item response theory with complex structures. Educational and Psychological Measurement, 73(2), 312-338.
[44] Muthén, L., & Muthén, B. (1998-2012). Mplus User’s Guide (Seventh Edition). Los Angeles, Ca: Muthén & Muthén.
[45] Muthén, B., du Toit, S. H. C., & Spisic, D. (1997). Robust inference using weighted least squares and quadratic estimating equations in latent variable modeling with categorical and continuous outcomes. Unpublished manuscript.
[46] West, S. G., Taylor, A. B., & Wu, W. (in press). Model fit and model selection in structural equation modeling. In R. H. Hoyle (Ed.), Handbook of Structural Equation Modeling. New York: Guilford Press.
[47] Joreskog, K., & Sorbom, D. (1996). User’s reference guide. Chicago, IL: Scientific Software International.
[48] Carroll, J. B. (1945). The effect of difficulty and chance success on correlations between items or between tests. Psychometrika, 10(1), 1-19.
[49] Wilson, D.,Wood, R., Gibbons, R., Schilling, S., Muraki, E., & Bock, R. D. (2003). TESTFACT: Test scoring and full information item factor analysis (Version 4.0). Lincoln-wood, IL: Scientific Software International.
[50] Reckase, M. (2009). Multidimensional item response theory (Vol. 150). New York: Springer.
[51] Sympson, J. B. (1978). A model for testing with multidimensional items. In D. J. Weiss (Ed.). Proceedings of the 1977 Computerized Adaptive Testing Conference (pp. 82-89). Minneapolis, MN: University of Minneapolis, Department of Psychology, Psychometric Methods Program.
[52] Kim, H. R. (1994). New techniques for the dimensionality assessment of standardized test data. Unpublished doctoral dissertation, University of Illinois at Urbana-Champaign.
[53] Zhang, J., & Stout, W. F.(1999). Conditional covariance structure of generalized compensatory multidimensional items. Psychometrika, 64(3), 129-152.
[54] Gessaroli, M. E., & De Champlain, A. F. (1996). Using an approximate chi-square statistic to test the number of dimensions underlying the responses to a set of items. Journal of Educational Measurement, 33(2), 157-179.
[55] Gessaroli, M. E., De Champlain, A. F., & Folske. (1997, March). Assessing dimensionality using a likelihood-ratio chi-square test based on a non-linear factor analysis of item response data. Paper presented at the annual meeting of the National Council on Measurement in Education, Chicago, IL.
[56] Fraser, C., & McDonald, R. P. (1988). NOHARM: Least squares item factor analysis. Multivariate Behavioral Research, 23(2), 267-269.
[57] Yuan, K. H., Chan, W., Marcoulides, G. A., & Bentler, P. M. (2016). Assessing structural equation models by equivalence testing with adjusted fit indexes. Structural Equation Modeling: A Multidisciplinary Journal, 23(3), 319-330.
[58] Marcoulides, K. M., & Yuan, K. H. (2017). New ways to evaluate goodness of fit: A note on using equivalence testing to assess structural equation models. Structural Equation Modeling: A Multidisciplinary Journal, 24(1), 148-153.
[59] Blömeke, S., Dunekacke, S., & Jenßen, L. (2017). Cognitive, educational and psychological determinants of prospective preschool teachers’ beliefs. European Early Childhood Education Research Journal, 1-19.
[60] Campbell, P., Hope, K., & Dunn, K. M. (2017). The pain, depression, disability pathway in those with low back pain: a moderation analysis of health locus of control. Journal of Pain Research, 10, 2331-2339.
[61] Cougle, J. R., Summers, B. J., Allan, N. P., Dillon, K. H., Smith, H. L., Okey, S. A., & Harvey, A. M. (2017). Hostile interpretation training for individuals with alcohol use disorder and elevated trait anger: a controlled trial of a web-based intervention. Behaviour Research and Therapy, 99, 57-66.
[62] Drinkwater, K., Denovan, A., Dagnall, N., & Parker, A. (2017). An assessment of the dimensionality and factorial structure of the revised paranormal belief scale. Frontiers in Psychology, 8, 1693.
[63] Firmin, R. L., Lysaker, P. H., McGrew, J. H., Minor, K. S., Luther, L., & Salyers, M. P. (2017). The Stigma Resistance Scale: A multi-sample validation of a new instrument to assess mental illness stigma resistance. Psychiatry Research, 258, 37-43.
[64] Govender, K., Cowden, R. G., Asante, K. O., George, G., & Reardon, C. (2017). Validation of the child and youth resilience measure among South African adolescents. PloS one, 12(10), e0185815.
[65] Heene, M., Hilbert, S., Draxler, C., Ziegler, M., & Bühner, M. (2011). Masking misfit in confirmatory factor analysis by increasing unique variances: a cautionary note on the usefulness of cutoff values of fit indices. Psychological Methods, 16(3), 319.
[66] Luo, Y., & Al-Harbi, K. (2016). The utility of the bifactor method for unidimensionality assessment when other methods disagree: an empirical illustration. SAGE Open, 6(4), 2158244016674513.
[67] Reise, S. P. (2012). The rediscovery of bifactor measurement models. Multivariate Behavioral Research, 47(5), 667-696.
[68] Jorgensen, T. D., Kite, B. A., Chen, P. Y., & Short, S. D. (2017). Permutation randomization methods for testing measurement equivalence and detecting differential item functioning in multiple-group confirmatory factor analysis. Psychological Methods, 23(4), 708-728.
[69] Kite, B. A., Jorgensen, T. D., & Chen, P. Y. (2018). Random permutation testing Applied to measurement invariance testing with ordered-categorical indicators. Structural Equation Modeling: A Multidisciplinary Journal, 25(4), 573-587.
Refbacks
- There are currently no refbacks.